Serviços Personalizados
Journal
Artigo
Links relacionados
Compartilhar
Ciencias Psicológicas
versão impressa ISSN 1688-4094versão On-line ISSN 1688-4221
Cienc. Psicol. vol.17 no.2 Montevideo dez. 2023 Epub 01-Dez-2023
https://doi.org/10.22235/cp.v17i2.3193
Original Articles
Satisfaction with online teaching in university students: Structural analysis of a scale
1 Universidad Científica del Sur, Perú
2 Universidad San Ignacio de Loyola, Perú
3 Universidad Privada Norbert Wiener, Perú, sdominguezmpcs@gmail.com
The aim of this research study was to analyze the internal structure and reliability of the Student Satisfaction Survey (SSS) in Peruvian university students. A total of 458 students participated (women = 69.9 %; M age = 27.76 years; SD age = 4.41 years). The SSS was studied under confirmatory factor analysis (CFA) and exploratory structural equation modeling (ESEM). Regard the results, the original five-dimensional model obtained favorable fit indexes with ESEM, but the dimensions student-teacher interactions and student-student interactions overlap each other, so it was valued as a four-dimensional model that presented better psychometric evidence. Regarding reliability, an acceptable order of magnitudes was observed, both at the level of scores and construct. It can be concluded that the SSS has adequate psychometric properties.
Keywords: satisfaction with online teaching; higher education; distance education; validity; reliability
El objetivo de esta investigación fue analizar la estructura interna y confiabilidad de la Student Satisfaction Survey (SSS) en estudiantes universitarios peruanos. Participaron 458 estudiantes (mujeres = 69.9 %; M edad = 27.76 años; DE edad = 4.41 años). La SSS se estudió bajo el análisis factorial confirmatorio (AFC) y el modelamiento exploratorio de ecuaciones estructurales (ESEM). Respecto a los resultados, el modelo original de cinco dimensiones obtuvo índices de ajuste favorables con ESEM, pero las dimensiones interacciones alumno-profesor e interacciones alumno-alumno se superponen entre sí, por lo que se valoró un modelo de cuatro dimensiones que presentó mejores evidencias psicométricas. La confiabilidad de las puntuaciones y de constructo presenta magnitudes aceptables. Se concluye que el SSS cuenta con propiedades psicométricas adecuadas.
Palabras clave: internacionalización; movilidad estudiantil; adultez emergente; identidad profesional
O objetivo deste estudo foi analisar a estrutura interna e a confiabilidade da Student Satisfaction Survey (SSS) em estudantes universitários peruanos. Participaram 458 estudantes (mulheres = 69,9 %; M idade = 27,76 anos; DP idade = 4,41 anos). O SSS foi estudado por meio de análise fatorial confirmatória (CFA) e modelação exploratória de equações estruturais (ESEM). Quanto aos resultados, o modelo original de cinco dimensões obteve índices de ajuste favoráveis com ESEM, mas as interações entre as dimensões aluno-professor e aluno-aluno se sobrepõem, por isso, foi analisado um modelo quatro dimensões que apresentou melhor evidência psicométrica. A confiabilidade das pontuações e de construto apresentaram magnitudes aceitáveis. Conclui-se que o SSS possui propriedades psicométricas adequadas.
Palavras-chave: satisfação com ensino online; ensino superior; educação a distância; validade; confiabilidade
The COVID-19 pandemic introduced abrupt changes in different areas of people's lives, and one of those that suffered the direct impact was the field of education. In this sense, the managers of educational institutions had to quickly adapt to the new demands to provide alternatives that guarantee the continuity of the educational processes. In this way, the online teaching modality was adopted by universities and all educational and administrative processes migrated to a digital interface.
Even before the pandemic, online teaching had already gradually gained greater prominence in the panorama of Peruvian higher education, in line with new pedagogical trends linked to the integration of information and communications technologies (TIC, in Spanish; Dominguez-Lara et al., 2022). However, doubts about the quality of the educational processes in online teaching were expressed through the results of the licensing and accreditation process of educational quality by the National Superintendence of Higher Education (SUNEDU, in Spanish), which is the governing body of evaluation of universities within the framework of the university reform in Peru. As a product of the aforementioned process, the operating license was denied to 48 out of the 140 universities that submitted to the evaluation process because they failed to demonstrate compliance with basic quality conditions (Benites, 2021), and many of these universities offered online teaching programs. In this way, the effects of the pandemic in the Peruvian educational field emerged in a context in which the university reform was underway and revealed a series of institutional problems, where online teaching was not a priority for higher education institutions.
In this scenario, universities faced many challenges for developing online education during the State of Health Emergency, and after it. Thus, some of the obstacles were related to the lack of access to technological devices or a stable internet connection by students and professors (Álvarez et al., 2020), while other limitations were associated with the skills required to the educational actors. On the one hand, the students were required to assume a more active and autonomous role in their own learning process, which, combined with the stress of a pandemic context, made them more emotionally vulnerable (Moreta-Herrera et al., 2022); and on the other hand, professors were forced to use and master TIC quickly, integrating them into their instructional activities after a brief training, and sometimes intuitively.
Despite these drawbacks, the effects of the pandemic made it possible to reflect on new ways of learning and teaching, in line with technological advances, and identifying a valuable opportunity for pedagogical reinvention and modernization of the university (Watermeyer et al., 2020). All this because many university students prefer online education motivated by the facilities it offers when articulating academic, work and family life (Waters & Russell, 2016), being an effective mechanism to shorten the gaps in access to higher education. (Kong et al., 2017). Therefore, universities must generate quality online education proposals and prepare students and their professors for a world integrated with technology (Sánchez-González & Castro-Higueras, 2022), given that blended learning (b-learning) combines both aspects, that is, face-to-face and online activities (Eryilmaz, 2015).
In this scenario, it is important to know the perspective of the university students since it has been shown that the success of an online education program is associated with their satisfaction (Kang & Park, 2022; Pham et al., 2019; Teo, 2010). This aspect is crucial to effective learning and is directly related to academic performance, retention, motivation and commitment to learning (Basith et al., 2020; Pham et al., 2019; Teo, 2010; Ye et al., 2022), aspects which, in turn, are associated with greater student autonomy (Vergara-Morales et al., 2022). For this reason, educational managers have the obligation to systematically evaluate learners’ satisfaction with educational processes as it is known that the university is an environment that generates various challenges for the student, whether at an academic, social, emotional and institutional level (Gravini-Donado et al., 2021).
From a classical perspective, user satisfaction is defined as an indicator of the distance between a comparison standard and the perceived performance of the good or service being evaluated (Oliver, 1980). In the academic field, student satisfaction refers to the value judgment about the fulfillment of their expectations, needs and demands during their educational experience (Bernard et al., 2009), although it has also been defined as the short-term attitude that is produced by the evaluation of your experience with the educational service received (Onditi & Wechuli, 2017).
Therefore, the study of student satisfaction in online learning environments is of growing interest because it influences the effectiveness of teaching and the development of instructional materials (Khan & Iqbal, 2016), mainly because its dynamics are different from that of face-to-face learning and must be valued according to those characteristics. Considering this will allow us to have useful information to design new online subjects and guide the improvement of teaching performance, as well as the learning content and the general quality of academic programs. This is relevant because, despite the advantages of online teaching (elimination of physical distances, time flexibility, among others), some limitations were identified (interpersonal communication problems, little cooperation from professors or virtual tutors, absence of direct contact, among others) that make it difficult for students to adapt (Díaz et al., 2013) and, consequently, harm academic performance and encourage dropout.
Similarly to face-to-face education, online education, is based on the interactive processes between participants. Thus, Moore and Kearsley (2005) point out that the effectiveness of teaching-learning will depend on the nature of this interaction and how it could be favored through a technological means (Moore, 2007). In this way, Moore (1993, 1997) describes a set of relations that also appear in online education when students and professors are distanced by space and time, highlighting three types of interactions for effective learning.
The first interaction is learner-content, referring to the students’ relations with the contents of the modules or learning units, the lessons and learning activities of the subjects, including readings, projects, videos, websites, among others, which lead to changes in understanding, perception and cognitive structure and significantly influence satisfaction with online teaching (Kuo, 2014).
The second interaction is learner-instructor, which implies a bidirectional relation between the student and the instructor whose function is to receive and give feedback, clarifying content and clearing doubts through fluid communication that facilitates and motivates learning (Yılmaz & Karataş, 2017), and is a significant predictor of satisfaction in synchronous classes (Kuo et al., 2014).
The third interaction is learner-learner, which refers to the bidirectional relation between students in order to share and learn cooperatively through different means such as discussion forums, emails or social networks, thus creating a collaboration between peers (Moore, 1993). This interaction is cognitive and social in nature and is important because it creates a sense of community (Shackelford & Maxwell, 2012) and enhances learning.
A fourth type of interaction is the so-called learner-technology interaction, proposed after the original three (Hanna et al., 2000; Palloff & Pratt, 2001), and refers to the communication between the student and the learning virtual environment, which implies knowing how to use virtual tools, as well as having the appropriate technological skills. This type of interaction focuses on the student's relations with the technological means and devices necessary to develop the educational program, considering aspects like the comfort and functionality of tools such as laptops, Internet, software or educational platforms, among others (Strachota, 2003).
The available evidence indicates that these types of interaction are relevant to student satisfaction and performance (Alqurashi, 2019; Basith et al., 2020; Kuo et al., 2013), highlighting some aspects associated with online teaching, such as: the design and content of the subjects, information accessibility on the virtual platform, the ease of interaction with the professor (Martín-Rodríguez et al., 2015), the interactions between students, administrative management and academic activities related to the procedural contents of the subjects (Nortvig et al., 2018). Furthermore, different studies emphasize students' preference for the synchronous modality of class delivery, which provides the opportunity to ask questions, debate and reflect in real time, complemented by asynchronous access to subject information and to the class recordings (Amir et al., 2020; Chung et al., 2020; Ramo et al., 2021).
According to the panorama presented, the evaluation of interactions in online learning environments and satisfaction with them is important, although the measurement instruments available present some flaws or methodological limitations that prevent valid and reliable use in certain aspects. For example, some instruments present only reliability reports (e.g., Baturay, 2011) and in other works this indicator is complemented only with expert opinion (e.g., Wei et al., 2015), but do not have an analysis of the internal structure of the instrument that allows the constructs to be differentiated from a factor-analytical perspective.
There are other instruments that do not present the procedural omissions of the previously mentioned studies. For example, a recently created scale (Yılmaz & Karataş, 2017) bases its hypothetical internal structure on Moore's approach (1993), but the methodological decisions in its construction are questionable since the use of principal components analysis overestimates the magnitude of factor loadings and deciding the number of factors using the Eigen value greater than unity criterion suggests extracting a greater than optimal number of factors (Lloret-Segura et al., 2014). Furthermore, the use of the same sample for both exploratory and confirmatory analysis is a not recommended practice because it provides inconclusive results (Pérez-Gil et al., 2000).
Another instrument available is the Student Satisfaction Survey (SSS; Strachota, 2003, 2006), which evaluates the dialogic components of interactions in the teaching-learning process based on the approaches of Moore and Kearsley (2005), complemented with the interaction between the learner and the technology and a dimension of general satisfaction. Among the advantages offered by the use of the SSS is that it can be applicable to all educational levels of higher education from a multidimensional theoretical perspective without including a large number of items. The SSS presents adequate psychometric properties, including validity evidence based on item content and an analysis of its internal structure through factor analysis, although under an exploratory approach.
Numerous works have used the SSS to directly measure dialogic interactions based on student satisfaction, both in online learning environments and in mixed environments (Mbwesa, 2014; Mohamed, 2021; Torrado & Blanca, 2022). For example, in research conducted with a university population in online and blended learning, it was measured how interactions in blended and online learning environments affected learning outcomes measured by student satisfaction and grades. The findings showed that the interaction that affected the learning results was the learner-content dyad, and the importance of the learner-instructor and learner-learner interaction in online learning environments was also highlighted (Ekwunife-Orakwue & Teng, 2014). In another study, the relations between academic self-efficacy, computer self-efficacy, previous experience and satisfaction with online learning were investigated, and a significant direct relation was found between academic self-efficacy and satisfaction with online learning (Jan, 2015). On the other hand, the influence of transactional dialogic interaction on learners’ satisfaction in a multi-institutional mixed learning environment was analyzed, and significantly positive effects of transactional interaction on satisfaction in mixed learning environments were found (Best & Conceição, 2017).
In this sense, the purpose of this work was to analyze the psychometric properties of the SSS in Peruvian university students in an online teaching context. On the one hand, the internal structure was examined using the exploratory structural equation modeling (ESEM) and on the other hand, the internal consistency of the scale was studied. This study is justified at a theoretical level because it will help understand the satisfaction structure with online teaching in Peruvian students given that the approach to this construct is emerging in Peru, and despite the fact that there are some pre-pandemic studies (e.g., Vásquez -Pajuelo, 2019), the psychometric properties of the instruments used are not clearly presented, which it does not allow satisfactory conclusions to be obtained. Likewise, at a practical level, institutions will be provided with an instrument that evaluates the dialogic interactions involved in the online teaching-learning process and that provides useful information on the areas that need to be improved through the design of learning environments that allow taking advantage of the available resources (Hanson et al., 2016; Magadán-Díaz & Rivas-García, 2022).
Finally, at a methodological level, although the validity evidence obtained in pioneering studies under an exploratory approach represents a good starting point (Strachota, 2003, 2006), it is necessary to analyze the scale under contemporary approaches that provide more information such as the ESEM (Asparouhov & Muthén, 2009). The ESEM provides the usual fit indices to evaluate the model in a similar way to the confirmatory factor analysis, but it also provides a complete estimate of the factor loadings (main and secondary ones) in the same way as the exploratory factor analysis, in addition to providing a more accurate estimate of the interfactor correlations (Asparouhov & Muthén, 2009), which would improve the understanding of this construct. However, although some works use confirmatory analysis (e.g., Yılmaz & Karataş, 2017), this approach assumes that the items only receive influence from their theoretical factor, leaving aside the other factors involved in the measurement model, which would represent an inconvenience when carrying out the analyzes (Marsh et al., 2014), since measures of complex constructs (such as satisfaction) usually have items with cross-loadings on other factors, and if they are not specified, even insignificant factor loadings (≈ .10) will negatively affect the model (Asparouhov et al., 2015).
Method
Design
This is an instrumental design (Ato et al., 2013) oriented to the analysis of psychometric properties of the Student Satisfaction Survey (Strachota, 2006).
Participants
458 Peruvian university students (69.9 % women; 31.1 % men) from various university degree courses of private institutions participated. The age ranged between 17 and 56 years old (M = 27.76; SD = 4.41), the majority were single (90.6 %), and more than half used the Internet more than 20 hours a week (52.2 %), while only 4.4 % did it less than five hours a week. The type of sampling was chosen for convenience since it considered accessibility and availability at a given time or the willingness to participate (Etikan, 2016) given the restrictions in force in Peru due to confinement during a health emergency.
Instrument
Student Satisfaction Survey (SSS;Strachota, 2006). This is a 25-item self-report scale with four response options (from completely disagree to completely agree) that evaluates general satisfaction with online teaching (e.g., “I would like to take other courses with the same learning environment”), as well as with different dimensions of interaction within that teaching context such as learner-content interactions (e.g., “The assignments or projects in those courses have facilitated my learning”), learner-instructor interactions (e.g., “I have received timely comments from my professors”) , learner-learner interactions (e.g., “In the courses I have been able to share my point of view with other students”), and learner-technology interactions (e.g., “Computers are of great help for learning”).
Procedure
The data was collected within the framework of a project focused on satisfaction with online education during the COVID-19 pandemic and was developed according to ethical recommendations of the American Psychological Association and the Declaration of Helsinki.
Authorization was requested from the creator to translate the instrument, which was done based on specialized literature (Muñiz et al., 2013). The first stage consisted of the translation from English to Spanish. It was then given to ten psychology students who assessed the clarity of the items and there were no problems understanding their content.
A Google forms link was sent to students between June and August 2021. The form contained the informed consent that had the title and description of the study, as well as the voluntary and anonymous nature of participation, which could be withdrawn whenever they wanted, and the confidential treatment of the data.
Data analysis
The five-factor original structure was analyzed using confirmatory factor analysis (CFA) and ESEM (Asparouhov & Muthén, 2009) because there are no studies that provide other measurement models. The analysis was carried out with the Mplus version 7 program (Muthén & Muthén, 1998-2015).
Skewness and kurtosis values between -2 and +2 would indicate a distribution approximate to the univariate normality of the items (Gravetter & Wallnau, 2014), and the multivariate normality with the Mardia coefficient (G2 < 70). Regarding the structural analysis, the WLSMV estimation method was used since it is oriented to ordinal items (Li, 2016a, 2016b), and based on the polychoric matrix correlation. The model analyzed with CFA and ESEM was assessed with the CFI (> .90; McDonald & Ho, 2002), the RMSEA (< .08; Browne & Cudeck, 1993), also considering the upper limit of its confidence interval (<. 10; West et al., 2012), and the WRMR (< 1; DiStefano et al., 2018). In the same way, in both the CFA and the ESEM, the convergent internal validity was analyzed with the average variance extracted (AVE > .37; Rubia, 2019) and with the magnitude of the factor loadings (λ >. 60; Dominguez-Lara, 2018a), as well as the discriminant internal validity if the square root of the AVE (√AVE) is greater than the interfactor correlation (ϕ) between two dimensions.
Regarding the CFA, interfactor correlations greater than .90 suggest factor redundancy (Brown, 2015). In relation to the ESEM, the oblique target rotation (ε = .05; Asparouhov & Muthén, 2009) was used, which freely estimates the main and secondary factor loadings, which were specified as close to zero (~0), to finally calculate the factor simplicity index (FSI) to assess its relevance. In that sense, an FSI above .70 is expected, which means that the item is influenced by a single factor (Lara et al., 2021).
On the other hand, the reliability of the scores (α > .70; Ponterotto & Charter, 2009) and of the construct (ω > .70; Hunsley & Marsh, 2008) was estimated, and whether the difference between coefficients is less than |. 06| (Δω-α) is not considered significant (Gignac et al., 2007). In this way, and given that it is desirable that the results of the SSS be configured as a profile, the reliability of the difference between two scores (ρ d ) was analyzed, which examines the degree to which the difference between two scores is explained more by the true variance than the error variance, so acceptable values (> .70) would indicate that the profile configuration provides relevant information (Dominguez-Lara, 2018b; Muñiz, 2003).
Results
The items present a magnitude of skewness and kurtosis that allow a reasonable approximation to univariate normality (Table 1), but not to multivariate normality (G2 = 291.775).
Table 1: Descriptive statistics of the items of the Student Satisfaction Survey
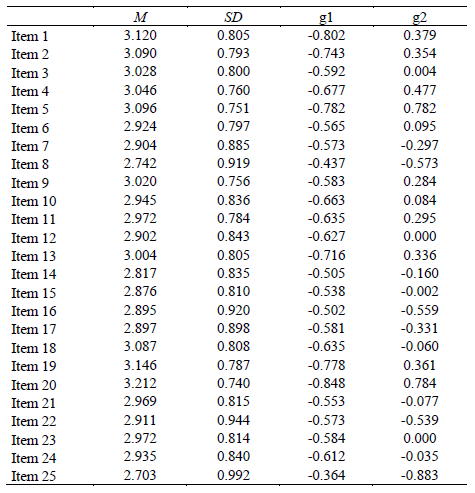
Note: M: Mean; SD: Standard Deviation; g1: skewness; g2: kurtosis.
The evaluation of the five-factor model provides favorable fit indices, both for the CFA approach (CFI = .959; RMSEA = .074, CI 90 % .069, .079; WRMR = 1.217) and for the ESEM (CFI = .977; RMSEA = .067, CI 90 % .061, .073; WRMR = 0.672), as well as mostly adequate factor loadings (> .50). Nevertheless, in the CFA a high interfactor correlation is observed between the dimensions learner-instructor interactions and learner-learner interactions (> .90), which in turn exceeds the root of the AVE of the two factors, indicating absence of discriminant internal validity. This situation stands out in the analysis with the ESEM approach in which four of the five items of the dimension learner-instructor interactions present factorial complexity since the dimension learner-learner interactions also influences them significantly, and item six (“In the courses, the professors have been active members of the discussion groups that offer guidance to our discussions”) has factorial complexity with the dimension learner-content interactions (Table 2). In that case, the dimensions involved were merged and analyzed again using ESEM.
Table 2: CFA and ESEM of the Student Satisfaction Survey: five-factor model
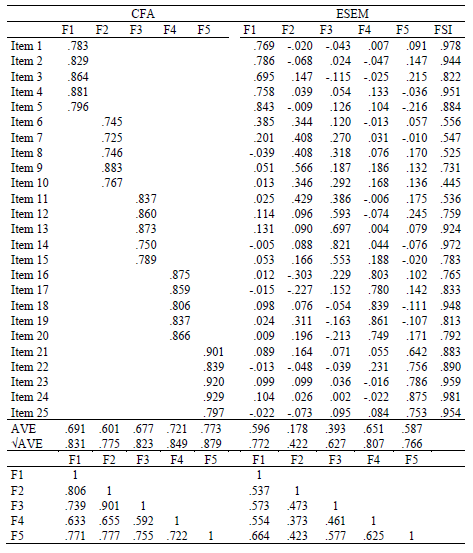
Note: F1: learner-content interactions; F2: learner-instructor interactions; F3: learner-learner interactions; F4: learner-technology interactions; F5: general satisfaction; FSI: Factor Simplicity Index; AVE: Average Variance Extracted.
In this way, the fit indices of the four-dimensional model were adequate (CFI = .962; RMSEA = .081, CI 90 % .075, .087; WRMR = 0.895), although item 6 maintained complexity with the dimension learner-content interactions, and item 14 (“In the courses I have received timely comments from other students”) was established as a Heywood case (λ > 1). By eliminating the two mentioned items, the fit improved (CFI = .968; RMSEA = .081, CI 90 % .075, .088; WRMR = 0.849), observing adequate factor loadings (λ > .60), sufficient factorial simplicity (FSI > .70), convergent internal validity (AVE > .50) and discriminant internal validity (√AVE > ϕ) (Table 3).
Table 3: ESEM and reliability of the Student Satisfaction Survey: four-factor model
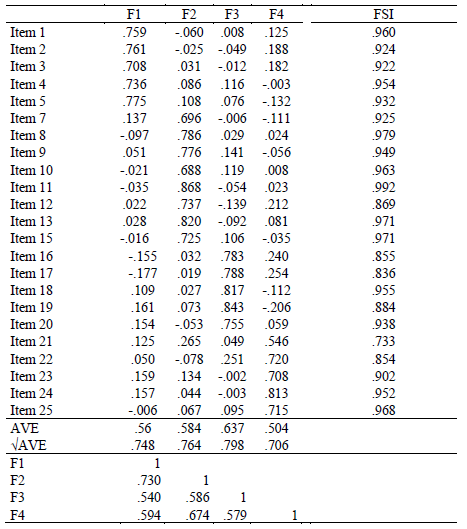
Note: F1: learner-content interactions (items 1-5); F2: learners-instructor interactions (items 7-13, 15); F3: learner-technology interactions (items 16-20); F4: general satisfaction (items 21-25); FSI: Factor Simplicity Index; AVE: Average Variance Extracted.
With respect to reliability, acceptable magnitudes are seen (> .80), while the reliability of the differences indicates that the profile resulting from the evaluation with the SSS is interpretable (Table 4).
Discussion
Considering that online teaching in the university environment is increasingly common, the measurement of student satisfaction emerges as a priority task to assess the effectiveness of these educational processes since it is a complex construct that involves many factors, such as communication, student participation in online discussions, flexibility, workload, technological support and professor’s pedagogical skills, among others. Therefore, it is crucial to have measurement instruments that are appropriate to each cultural context to measure it, and therefore, the purpose of this research was to analyze the internal structure and reliability of the Student Satisfaction Survey (SSS) in Peruvian university students.
Although the analysis carried out on the internal structure of the SSS indicates that most of the dimensions are robust, an overlap is suggested between the original dimensions called learner-instructor interactions and learner-learner interactions, both with the CFA, by presenting an interfactor high correlation, as with the ESEM, due to the high factorial complexity observed. This implies that interactions with professors and students are perceived as part of the same situation, that is, as an interaction within the class context, which supports a satisfactory experience with the content (Kuo et al., 2014)), thus a sense of community among students (Shackelford & Maxwell, 2012), due to the importance of interaction between class actors (learners and professor) in online classes (Ekwunife-Orakwue & Teng, 2014).
Despite the scarcity of studies that explore the psychometric properties of the SSS in Spanish-speaking countries, the results found are comparable with those of Torrado & Blanca (2022), in which favorable evidence was found for the five-factor model, although some important indicators that inform the differentiation of the factors are not reported, such as the values of the interfactor correlations and the values of the AVE. In any case, the findings of the present study correspond to the way in which the types of interaction are manifested in synchronous online education and can be interpreted from the theory of transactional distance (Moore, 1993, 1997, 2007). In effect, the interaction that occurs between students in online teaching is different from that which occurs face-to-face (Thurmond & Wambach, 2004), especially in the synchronous modality, in which said interaction depends largely on the learner-instructor interaction, as teamwork will be carried out whenever the professor develops a teaching methodology that promotes collaborative learning, providing opportunities for students to exchange information to carry out academic tasks and fostering a sense of learning community (Alqurashi, 2019; Basith et al., 2020; Shackelford & Maxwell, 2012; Thurmond & Wambach, 2004). On the other hand, unlike what happens in a face-to-face classroom, the learner-instructor interaction in the virtual environment no longer takes center stage and the instructor becomes a facilitator of learning, hence the interactions between students and the interaction with the instructor are perceived as part of the same component. Previous studies have already pointed out how these two types of interaction differ from others because they significantly strengthen the learners’ sense of belonging with respect to their learning community (Luo et al., 2017).
Likewise, during the process of analyzing the internal structure of the SSS, two items were eliminated. The first of these was item 6 (“In the courses, professors have been active members of the discussion groups that offer guidance to our discussions”), probably because due to the migration to virtual environments and the predominance of online classes, the number per classroom doubled or tripled, which would have prevented more personalized attention from professors. For its part, item 14 (“In the courses I have received timely comments from other students”) is probably not representative because there are currently other means to communicate with other students (e.g., social networks), unlike the time in which the scale was created with communication between students focused on the platform used by the institution or the synchronous class.
With respect to reliability, the alpha and omega coefficients reached adequate values (Hunsley & Marsh, 2008; Ponterotto & Charter, 2009). Therefore, it is suggested that the SSS is a reliable instrument, just like reported in previous studies that evaluate satisfaction with online teaching (Strachota, 2006; Torrado & Blanca, 2022). Furthermore, reliability of the difference between two scores provides evidence that the SSS can configure an integrated assessment profile of satisfaction with teaching and not only report separately on each dimension of the construct (Dominguez-Lara, 2018b).
Regarding the practical implications of the study, the SSS can be used to measure the students’ satisfaction who study online and based on the results, useful information can be obtained about the strengths and weaknesses of said educational programs in a way that guidelines and improvement strategies that promote an optimal learning environment can be implemented. In the same way, the scores derived from the SSS can be considered as indicators of the effectiveness of online courses, providing more information for the decision-making of educational managers (Chen & Tat Yao, 2016; Palmer & Holt, 2009).
However, despite the implications and strengths of this study, it is not exempt from some limitations that should be mentioned. Firstly, the type of sampling was convenience, and therefore, the results may not be representative of the entire population. Secondly, the study was limited to the response of the self-report scale, which could raise potential problems related to social desirability bias, but as it was an anonymous and online evaluation, this bias is likely to be attenuated (Larson, 2018). Thirdly, the scaling of the items into four response options could be reviewed in subsequent studies given that it could sometimes affect the psychometric properties (Donnellan et al., 2023). Finally, the convergent and predictive validity of the scale was not evaluated. Despite these limitations, the study provides a psychometric basis regarding the internal structure of the SS for an adequate measurement of satisfaction with online teaching.
Thus, it is recommended to replicate the study to explore whether the original dimensions that reflect the interaction between learners and professors are really redundant, and to analyze whether the amount of options proposed by the original author (four) provides better psychometric parameters than another number of alternatives. Likewise, it is recommended that future research use probability sampling techniques to obtain a more precise estimate. Future studies should examine the association of SSS with other variables with the aim of expanding the validity evidence.
It is concluded that the SSS presents a four-dimensional structure and adequate reliability indicators.
REFERENCES
Alqurashi, E. (2019). Predicting student satisfaction and perceived learning within online learning environments. Distance Education, 40(1), 133-148. https://doi.org/10.1080/01587919.2018.1553562 [ Links ]
Álvarez, M., Gardyn, N., Iardelevsky, A., & Rebello, G. (2020). Segregación educativa en tiempos de pandemia: Balance de las acciones iniciales durante el aislamiento social por el Covid-19 en Argentina. Revista Internacional de Educación para la Justicia Social, 9(3), 25-43. [ Links ]
Amir, L. R., Tanti, I., Maharani, D. A., Wimardhani, Y. S., Julia, V., Sulijaya, B., & Puspitawati, R. (2020). Student perspective of classroom and distance learning during COVID-19 pandemic in the undergraduate dental study program Universitas Indonesia. BMC Medical Education, 20(1), 1-8. https://doi.org/10.1186/s12909-020-02312-0 [ Links ]
Asparouhov, T., & Muthén, B. O. (2009). Exploratory structural equation modeling. Structural Equation Modeling, 16(3), 397-438. http://doi.org/10.1080/10705510903008204 [ Links ]
Asparouhov, T., Muthen, B., & Morin, A. J. S. (2015). Bayesian structural equation modeling with cross-loadings and residual covariances. Journal of Management, 41(6), 1561-1577. http://doi.org/10.1177/0149206315591075 [ Links ]
Ato, M., López-García, J. J., & Benavente, A. (2013). Un sistema de clasificación de los diseños de investigación en psicología. Anales de Psicología, 29(3), 1038-1059. https://doi.org/10.6018/analesps.29.3.178511 [ Links ]
Basith, A., Rosmaiyadi, R., Triani, S. N., & Fitri, F. (2020). Investigation of online learning satisfaction during COVID 19: In relation to academic achievement. Journal of Educational Science and Technology, 6, 265-275. https://doi.org/10.26858/est.v1i1.14803 [ Links ]
Baturay, M. H. (2011). Relationships among sense of classroom community, perceived cognitive learning and satisfaction of students at an e-learning course. Interactive Learning Environments, 19(5), 563-575. https://doi.org/10.1080/10494821003644029 [ Links ]
Benites, R. (2021). La educación superior universitaria en el Perú post-pandemia. Políticas y Debates públicos. https://repositorio.pucp.edu.pe/index/handle/123456789/176597 [ Links ]
Bernard, R. M., Abrami, P. C., Borokhovski, E., Wade, C. A., Tamim, R. M., Surkes, M. A., & Bethel, E. C. (2009). A meta-analysis of three types of interaction treatments in distance education. Review of Educational Research, 79(3), 1243-1289. https://doi.org/10.3102/0034654309333844 [ Links ]
Best, B., & Conceição, S. C. O. (2017). Transactional distance dialogic interactions and student satisfaction in a multi-institutional blended learning environment. European Journal of Open, Distance and e-Learning, 20(1), 138-152. [ Links ]
Brown, T. A. (2015). Confirmatory factor analysis for applied research (2a ed.). Guilford Press. [ Links ]
Browne, M. W., & Cudeck, R. (1993). Alternative ways of assessing model fit. En K. A. Bollen & J. S. Long (Eds.), Testing structural equation models (pp. 445-455). Sage. [ Links ]
Chen, W. S., & Tat Yao, A. Y. (2016). An empirical evaluation of critical factors influencing learner satisfaction in blended learning: a pilot study. Universal Journal of Educational Research, 4(7), 1667-1671. https://doi.org/10.13189/ujer.2016.040719 [ Links ]
Chung, E., Subramaniam, G., & Dass, L. C. (2020). Online learning readiness among university students in Malaysia amidst COVID19. Asian Journal of University Education, 16(2), 46-58. [ Links ]
Díaz, V. M., Urbano, E. R., & Berea, G. M. (2013). Ventajas e inconvenientes de la formación online. Revista Digital de Investigación en Docencia Universitaria, 7(1), 33-43. https://doi.org/10.19083/ridu.7.185 [ Links ]
DiStefano, C., Liu, J., Jiang, N., & Shi, D. (2018). Examination of the weighted root mean square residual: Evidence for trustworthiness? Structural Equation Modeling: A Multidisciplinary Journal, 25(3), 453-466. https://doi.org/10.1080/10705511.2017.1390394 [ Links ]
Dominguez-Lara, S. (2018a). Propuesta de puntos de corte para cargas factoriales: una perspectiva de confiabilidad de constructo. Enfermería Clínica, 28(6), 401-402. https://doi.org/10.1016/j.enfcli.2018.06.002 [ Links ]
Dominguez-Lara, S. (2018b). Reporte de las diferencias confiables en el perfil del ACE-III. Neurología, 33(2), 138-139. http://doi.org/10.1016/j.nrl.2016.02.022 [ Links ]
Dominguez-Lara, S., Gravini-Donado, M., Moreta-Herrera, R., Quistgaard-Alvarez, A., Barboza-Zelada, L. A., & De Taboada, L. (2022). Propiedades psicométricas del Student Adaptation to College Questionnaire - Educación Remota en estudiantes universitarios de primer año durante la pandemia. Campus Virtuales, 11(1), 81-93. https://doi.org/10.54988/cv.2022.1.965 [ Links ]
Donnellan, M. B., & Rakhshani, A. (2023). How does the number of response options impact the psychometric properties of the Rosenberg Self-Esteem Scale? Assessment, 30(6), 1737-1749. https://doi.org/10.1177/10731911221119532 [ Links ]
Ekwunife-Orakwue, K. C., & Teng, T. L. (2014). The impact of transactional distance dialogic interactions on student learning outcomes in online and blended environments. Computers & Education, 78, 414-427. https://doi.org/10.1016/j.compedu.2014.06.011 [ Links ]
Eryilmaz, M. (2015). The effectiveness of blended learning environments. Contemporary Issues in Education Research, 8(4), 251-256. https://doi.org/10.19030/cier.v8i4.9433 [ Links ]
Etikan, I. (2016). Comparison of Convenience Sampling and Purposive Sampling. American Journal of Theoretical and Applied Statistics, 5(1), 1. https://doi.org/10.11648/j.ajtas.20160501.11 [ Links ]
Gignac, G. E., Bates, T. C., & Jang, K. (2007). Implications relevant to CFA model misfit, reliability, and the Five Factor Model as measured by the NEO-FFI. Personality and Individual Differences, 43(5), 1051-1062. https://doi.org/10.1016/j.paid.2007.02.024 [ Links ]
Gravetter, F., & Wallnau, L. (2014). Essentials of Statistics for the Behavioral Sciences. Wadsworth. [ Links ]
Gravini-Donado, M. L., Mercado-Peñaloza, M., & Dominguez-Lara, S. (2021). College Adaptation Among Colombian Freshmen Students: Internal Structure of the Student Adaptation to College Questionnaire (SACQ). Journal of New Approaches in Educational Research, 10(2), 251-263. http://doi.org/ 10.7821/naer.2021.7.657 [ Links ]
Hanna, D. E., Glowacki-Dudka, M., & Runlee, S. (2000). 147 practical tips for teaching online groups. Atwood. [ Links ]
Hanson, J., Bangert, A., & Ruff, W. (2016). A validation study of the what’s my school mindset? Survey. Journal of Educational Issues, 2(2), 244-266. [ Links ]
Hunsley, J., & Marsh, E. J. (2008). Developing criteria for evidence-based assessment: An introduction to assessment that work. En J. Hunsley & E. J. Marsh (Eds.), A guide to assessments that work (pp. 3-14). Oxford University Press. [ Links ]
Jan, S. K. (2015). The relationships between academic self-efficacy, computer self-efficacy, prior experience, and satisfaction with online learning. American Journal of Distance Education, 29(1), 30-40. https://doi.org/10.1080/08923647.2015.994366 [ Links ]
Kang, D., & Park, M. J. (2022). Interaction and online courses for satisfactory university learning during the COVID-19 pandemic. The International Journal of Management Education, 20(3), 100678. https://doi.org/10.1016/j.ijme.2022.100678 [ Links ]
Khan, J., & Iqbal, M. J. (2016). Relationship between student satisfaction and academic achievement in distance education: a case study of AIOU Islamabad. FWU Journal of Social Sciences, 10(2), 137-145. [ Links ]
Kong, S. C., Looi, C. K., Chan, T. W., & Huang, R. (2017). Teacher development in Singapore, Hong Kong, Taiwan, and Beijing for e-learning in school education. Journal of Computers in Education, 4(1), 5-25. https://doi.org/10.1007/s40692-016-0062-5 [ Links ]
Kuo, Y. C. (2014). Accelerated online learning: Perceptions of interaction and learning outcomes among African American students. American Journal of Distance Education, 28(4), 241-252. https://doi.org/10.1080/08923647.2014.959334 [ Links ]
Kuo, Y. C., Belland, B. R., Schroder, K. E., & Walker, A. E. (2014). K-12 teachers’ perceptions of and their satisfaction with interaction type in blended learning environments. Distance Education, 35(3), 360-381. https://doi.org/10.1080/01587919.2015.955265 [ Links ]
Kuo, Y. C., Walker, A. E., Belland, B. R., & Schroder, K. E. E. (2013). A predictive study of student satisfaction in online education programs. International Review of Research in Open and Distributed Learning, 14(1), 16-39. https://doi.org/10.19173/irrodl.v14i1.1338 [ Links ]
Lara, L., Monje, M. F., Fuster-Villaseca, J., & Dominguez-Lara, S. (2021). Adaptación y validación del Big Five Inventory para estudiantes universitarios chilenos. Revista Mexicana de Psicología, 38(2), 83-94. [ Links ]
Larson, R. B. (2018). Controlling social desirability bias. International Journal of Market Research, 61(5), 534-547. http://doi.org/10.1177/1470785318805305 [ Links ]
Li, C. (2016a). Confirmatory factor analysis with ordinal data: Comparing robust maximum likelihood and diagonally weighted least squares. Behavioral Research Methods, 48, 936-949. https://doi.org/10.3758/s13428-015-0619-7 [ Links ]
Li, C. (2016b). The performance of ML, DWLS, and ULS estimation with robust corrections in structural equation models with ordinal variables. Psychological Methods, 21(3), 369-387. https://doi.org/10.1037/met0000093 [ Links ]
Lloret-Segura, S., Ferreres-Traver, A., Hernández-Baeza, A., & Tomás-Marco, I. (2014). El análisis factorial exploratorio de los ítems: una guía práctica, revisada y actualizada. Anales de psicología, 30(3), 1151-1169. http://doi.org/10.6018/analesps.30.3.199361 [ Links ]
Luo, N., Zhang, M., & Qi, D. (2017). Effects of different interactions on students’ sense of community in e-learning environment. Computers & Education, 115, 153-160. https://doi.org/10.1016/j.compedu.2017.08.006 [ Links ]
Magadán-Díaz, M., & Rivas-García, J. I. (2022). Gamificación del aula en la enseñanza superior online: el uso de Kahoot. Campus Virtuales, 11(1), 137-152. https://doi.org/10.54988/cv.2022.1.978 [ Links ]
Marsh, H. W., Morin, A. J. S., Parker, P. D., & Kaur, G. (2014). Exploratory structural equation modeling: An integration of the best features of exploratory and confirmatory factor analysis. Annual Review of Clinical Psychology, 10(1), 85-110. http://doi.org/10.1146/annurev-clinpsy-032813-153700 [ Links ]
Martín-Rodríguez, Ó., Fernández-Molina, J. C., Montero-Alonso, M. Á., & González-Gómez, F. (2015). The main components of satisfaction with e-learning. Technology, Pedagogy and Education, 24(2), 267-277. https://doi.org/10.1080/1475939X.2014.888370 [ Links ]
Mbwesa, J. K. (2014). Transactional distance as a predictor of perceived learner satisfaction in distance learning courses: A case study of bachelor of education arts program, University of Nairobi, Kenya. Journal of Education and Training Studies, 2(2), 176-188. https://doi.org/10.11114/jets.v2i2.291 [ Links ]
McDonald, R.P. & Ho, M.H.R. (2002). Principles and practice in reporting structural equation analyses. Psychological Methods, 7(1), 64-82. https://doi.org10.1037/1082-989X.7.1.64 [ Links ]
Mohamed, E., Ghaleb, A., & Abokresha, S. (2021). Satisfaction with online learning among Sohag University students. Journal of High Institute of Public Health, 51(2), 84-89. https://doi.org/10.21608/jhiph.2021.193888 [ Links ]
Moore, M. (1993). Three types of interaction. En K. Harry, M. John & D. Keegan (Eds.), Distance education theory (pp. 19-24). Routledge. [ Links ]
Moore, M. G. (1997). Theory of transactional distance. En D. Keegan (Ed.), Theoretical Principles of Distance Education (pp. 22-38). Routledge. [ Links ]
Moore, M. G. (2007). Theory of transactional distance. En M. G. Moore (Ed.). Handbook of distance education (pp. 89-101). Lawrence Erlbaum. [ Links ]
Moore, M., & Kearsley, G. (2005). Distance education: A system view. Thomson-Wadsworth. [ Links ]
Moreta-Herrera, R., Vaca-Quintana, D., Quistgaard-Álvarez, A., Merlyn-Sacoto, M.-F., & Dominguez-Lara, S. (2022). Análisis psicométrico de la Escala de Cansancio Emocional en estudiantes universitarios ecuatorianos durante el brote de COVID-19. Ciencias Psicológicas, 16(1), e-2755. https://doi.org/10.22235/cp.v16i1.2755 [ Links ]
Muñiz, J. (2003). Teoría clásica de los tests. Pirámide. [ Links ]
Muñiz, J., Elosua, P., & Hambleton, R. K. (2013). Directrices para la traducción y adaptación de los test: segunda edición. Psicothema, 25(2), 151-157. https://doi.org/10.7334/psicothema2013.24 [ Links ]
Muthén, L. K., & Muthén, B.O. (1998-2015). Mplus User’s Guide. Muthén & Muthén. [ Links ]
Nortvig, A. M., Petersen, A. K., & Balle, S. H. (2018). A Literature review of the factors influencing e‑learning and blended learning in relation to learning outcome, student satisfaction and engagement. Electronic Journal of E-learning, 16(1), 46-55. [ Links ]
Oliver, R. L. (1980). A cognitive model of the antecedents and consequences of satisfaction decision. Journal of Marketing Research, 17(4), 460-469. https://doi.org/10.1177/002224378001700405 [ Links ]
Onditi, E. O., & Wechuli, T. W. (2017). Service quality and student satisfaction in higher education institutions: A review of literature. International Journal of Scientific and Research Publications, 7(7), 328-335. [ Links ]
Palloff, R. M., & Pratt, K. (2001). Lessons from the cyberspace classroom. The realities of online teaching. Jossey-Bass. [ Links ]
Palmer, S. R., & Holt, D. M. (2009). Examining student satisfaction with wholly online learning. Journal of Computer Assisted Learning, 25(2), 101-113. https://doi.org/10.1111/j.1365-2729.2008.00294.x [ Links ]
Pérez-Gil, J. A., Chacón, S. & Moreno, R. (2000). Validez de constructo: el uso de análisis factorial exploratorio-confirmatorio para obtener evidencias de validez. Psicothema, 12, 442-446. [ Links ]
Pham, L., Limbu, Y. B., Bui, T. K., Nguyen, H. T., & Pham, H. T. (2019). Does e-learning service quality influence e-learning student satisfaction and loyalty? Evidence from Vietnam. International Journal of Educational Technology in Higher Education, 16(1), 1-26. https://doi.org/10.1186/s41239-019-0136-3 [ Links ]
Ponterotto, J., & Charter, R. (2009). Statistical extensions of Ponterotto and Ruckdeschel’s (2007) reliability matrix for estimating the adequacy of internal consistency coefficients. Perceptual and Motor Skills, 108(3), 878-886. https://doi.org/10.2466/PMS.108.3.878-886 [ Links ]
Ramo, N. L., Lin, M. A., Hald, E. S., & Huang-Saad, A. (2021). Synchronous vs. asynchronous vs. blended remote delivery of introduction to biomechanics course. Biomedical Engineering Education, 1, 61-66. https://doi.org/10.1007/s43683-020-00009-w [ Links ]
Rubia, J. M. (2019). Revisión de los criterios para validez convergente estimada a través de la VarianRubiaza Media Extraída. Psychologia, 13(2), 25-41. https://doi.org/10.21500/19002386.4119 [ Links ]
Sánchez-González, M., & Castro-Higueras, A. (2022). Mentorías para profesorado universitario ante la Covid-19: evaluación de un caso. Campus Virtuales, 11(1), 181-200. https://doi.org/10.54988/cv.2022.1.1000 [ Links ]
Shackelford, J. L., & Maxwell, M. (2012). Sense of community in graduate online education: Contribution of learner to learner interaction. The International Review of Research in Open and Distributed Learning, 13(4), 228-249. https://doi.org/10.19173/irrodl.v13i4.1339 [ Links ]
Strachota, E. (2003). Student satisfaction in online course: An analysis of the impact of learner-content, learner-instructor, learner-learner and learner-technology interaction (Disertación doctoral). University of Wisconsin- Milwaukee. [ Links ]
Strachota, E. (2006). The use of survey research to measure student satisfaction in online courses. University of Missouri-St. Louis. [ Links ]
Teo, T. (2010). A structural equation modelling of factors influencing student teachers’ satisfaction with e-learning. British Journal of Educational Technology, 41(6), 150-152. https://doi.org/10.1111/j.1467-8535.2010.01110.x [ Links ]
Thurmond, V., & Wambach, K. (2004). Understanding interactions in distance education: A review of the literature. International Journal of Instructional Technology and Distance Learning, 1(1), 9-26. [ Links ]
Torrado, M., & Blanca, M. J. (2022). Assessing satisfaction with online courses: Spanish version of the Learner Satisfaction Survey. Frontiers in Psychology, 13, 875929. https://doi.org/10.3389/fpsyg.2022.875929 [ Links ]
Vásquez-Pajuelo, L. (2019). Aprendizaje online: satisfacción de los universitarios con experiencia laboral. Review of Global Management, 5(2), 28-43. https://doi.org/10.19083/rgm.v5i2.1234 [ Links ]
Vergara-Morales, J., Rodríguez-Vera, M., & Del Valle, M. (2022). Evaluación de las propiedades psicométricas del Cuestionario de Autorregulación Académica (SRQ-A) en estudiantes universitarios chilenos. Ciencias Psicológicas, 16(2), e-2837. https://doi.org/10.22235/cp.v16i2.2837 [ Links ]
Watermeyer, R., Crick, T., Knight, C., & Goodall, J. (2020). COVID-19 and digital disruption in UK universities: afflictions and affordances of emergency online migration. Higher Education, 81(3), 623-641. https://doi.org/10.1007/s10734-020-00561-y [ Links ]
Waters, S., & Russell, W. (2016). Virtually ready? Pre-service teachers’ perceptions of a virtual internship experience. Research in Social Sciences and Technology, 1(1), 1-23. [ Links ]
Wei, H. C., Peng, H., & Chou, C. (2015). Can more interactivity improve learning achievement in an online course? Effects of college students’ perception and actual use of a course-management system on their learning achievement. Computers & Education, 83, 10-21. https://doi.org/10.1016/j.compedu.2014.12.013 [ Links ]
West, S. G., Taylor, A. B., & Wu, W. (2012). Model fit and model selection in structural equation modeling. En R. H. Hoyle (Ed.), Handbook of Structural Equation Modeling (pp. 209-231). Guilford. [ Links ]
Ye, J-H., Lee, Y-S., & He, Z. (2022). The relationship among expectancy belief, course satisfaction, learning effectiveness, and continuance intention in online courses of vocational-technical teachers college students. Frontiers in Psychology, 13, 904319. https://doi.org/10.3389/fpsyg.2022.904319 [ Links ]
Yılmaz, A., & Karataş, S. (2017). Development and validation of perceptions of online interaction scale. Interactive Learning Environments, 26(3), 337-354. http://doi.org/10.1080/10494820.2017.1333009 [ Links ]
How to cite: Manrique-Millones, D., Lingán-Huamán, S. K., & Dominguez-Lara, S. (2023). Satisfaction with online teaching in university students: Structural analysis of a scale. Ciencias Psicológicas, 17(2), e-3193. https://doi.org/10.22235/cp.v17i2.3193
Authors’ participation: a) Conception and design of the work; b) Data acquisition; c) Analysis and interpretation of data; d) Writing of the manuscript; e) Critical review of the manuscript. D. M. M. has contributed in a, b, d, e; S. K. L. H. in a, c, d, e; S. D. L. in a, b, c, d, e.
Received: January 31, 2023; Accepted: October 04, 2023